This chapter is also published as working paper:
Miörner J., Truffer B., Binz C., Heiberg J. & Yap X.-S. (2022) Guidebook for applying the Socio-Technical Configuration Analysis method. GEIST – Geography of Innovation and Sustainability Transitions 2022(1), GEIST Working Paper series.
Writing this guidebook was a truly collaborative effort over the past year and the development of the STCA methodology over the past four years is the co-creation of all authors listed here. J. Miörner coordinated the writing process of the guidebook and wrote substantial parts of chapters 2-7. B. Truffer acted as the lead author for the present introductory working paper with contributions from all authors. The remaining authors are listed in alphabetical order. J. Heiberg pioneered the original method development in the context of his PhD project (see Heiberg, 2022). Besides the guidebook, all authors contributed to developing and refining STCA as an innovative research method by conducting a wide variety of pioneering test cases. References to these early cases may be found across the guidebook.
The present chapter provides an introduction to the conceptual and methodological foundations of Socio-Technical Configuration Analysis (STCA). Socio-technical configurations are associations of technologies and institutions that get aligned with each other by actors to fulfil a societal function (e.g. provision of energy, food, water, transport, etc.). Institutions are defined as the regulative, normative or cognitive rules that structure human actions, e.g. preferences, values, regulations, standards, etc. (see Scott, 1995). For instance, cars can only be reliably operated if a supportive infrastructure (paved roads, gas stations, traffic lights) is available, if traffic codes exist that are respected, and if users are trained to operate the vehicles in compliance with these rules. Socio-technical configurations cannot be observed directly because many of the relevant rules are not codified anywhere. They can however be derived from analyzing narratives or practices of people. For instance, key actors will relate to the above dimensions when being asked to defend automobiles as the “best” way to provide passenger mobility services. Or when driving automobiles, users will be following the relevant codified and implicit rules (at least most of the time).
STCA enables the identification of key elements of socio-technical configurations from texts, in which actors comment on the appropriateness of specific technologies to solve problems or the rules around using these technologies, or in which activities of key stakeholders are reported on how they actually combine technical and social elements to achieve specific goals. At a fundamental level, STCA can thus be applied with two types of foci: i) the discursive focus derives socio-technical configurations from (reported or actual) statements of actors, in which they evaluate certain combinations of technological and institutional terms. ii) The substantive focus identifies concrete activities and/or institutional changes that actors induce in a given field. It is based on reports of actual activities of actors (‘events’), such as major investments, promotional activities, opening of new market segments, founding of research centres, etc., where both technologies and institutional aspects are mentioned. In the following, we will often refer to examples that were derived with a discursive focus, but the same analyses could equally well be carried out with a focus on substantive system reconfiguration dynamics as reported in texts.
Document stocks from where these texts are drawn may be collections of newspaper articles or professional magazines, but also expert interview transcripts, government session protocols, business reports, social media feeds, or any other consistent collection of texts, which elaborates on a specific technology and the context of its application, development or legitimation. Depending on the scope and coverage of these document stocks, STCA enables retracing socio-technical reconfiguration and transition dynamics across sectors, time and space.
STCA builds on and extends earlier text-based methodologies in innovation and transition studies, such as event analysis (with a substantive focus, see Hekkert et al. (2007)), or discourse analysis (with a discursive focus, see Hajer (1995)). Yet, STCA goes beyond these precedents by adopting an explicit configurational lens to actors and “concepts” derived from texts. This means that not only frequencies of coded terms are analyzed but also their topological relationships, which indicates how strongly these terms have been associated with each other in arguments or actions of specific actors. To that end, STCA builds on the family of social network analysis (SNA) methods (Wasserman & Faust 2012). However, interest is less on actual, material collaboration networks between actors and more on worldviews, narratives and practices that different actors share (like story lines used by incumbent regime actors to legitimize their strategies, compared to the respective story lines of niche actors). The procedural steps of STCA were originally inspired by the Discourse Network Analysis method (Leifeld, 2013) from political sciences. DNA enables identifying actor coalitions that express opinions in favor of or against specific policy proposals. STCA, in contrast, is less interested in policy processes or specific actor coalitions, but more in the socio-technical storylines that actors co-construct and take as templates for defending their actions. In order to distinguish collaborative networks among actors from the focus of STCA on similar world views, we use the term “actor congruence networks” in the following, referring to similar references to configurations shared by different actors, without necessarily engaging in mutual exchanges. The broader epistemological context in which STCA may be applied will mostly consist of mixed methods research designs (Goertz and Mahoney, 2012; Creswell, 2009) that are complemented with either qualitative or quantitative analyses. It furthermore aligns with the family of “complexity sciences” (Mitchell, 2009; Balland et al., 2022) and provides a novel methodological tool to enable configurational theorizing (Furnari et al., 2021; Abbott, 2001; Weber and Truffer, 2017).
This guidebook provides a step-by-step and hands-on introduction on how to select suitable document stocks, how to code texts, and how these codes may then be mapped to highlight topological relationships between codes. It is intended for newcomers from innovation and transition studies, as well as related fields in the social sciences such as geography, management studies, political sciences, or sociology. A more systematic introduction to STCA, its conceptual approach and empirical illustrations can be found in Heiberg et al. (2022), as well as in other publications of our research group (see list of publications and other resources under Resources). We first elaborate on the theoretical contexts in which socio-technical configurations, their dynamics and geographical variations play a key role and how they relate to conceptual frameworks from innovation and transition studies. Secondly, we specify the “theory of measurement” (Goertz, 2020) on how statements or actions by actors reported in the selected document stocks can be aggregated into coherent storylines or strategies, which may be interpreted as representing socio-technical configurations that are supported by more or less coherent collections of actors. Shifts over time of these networks can then be interpreted as depicting transition dynamics, comparisons across space as local variations of regime or innovation system structures. Third, we introduce a typology of ideal-type research problems that can be analyzed by applying STCA. Finally, we provide an overview on the generic steps that a researcher has to conduct when applying the method.
2. Socio-technical configurations: Frameworks and concepts
The origins of socio-technical thinking can be traced back to the 1980s when Evolutionary Economics (EE) and Science and Technology Studies (STS) started rejecting techno-determinism (Smith et al., 2010; Misa, 1998) or the treatment of technology as an exogenous variable in neo-classical economics (Romer, 1994; Nelson and Winter, 1982). Especially evolutionary economists started to embrace sociological approaches, conceptualizing technological change as dependent upon particular cognitive routines among professional communities that channel innovation into specific trajectories (so-called technological regimes, see Dosi et al. (1988)), but also on the socially constructed selection environment of markets and regulatory structures, into which these communities are embedded (Nelson and Winter, 1982). They furthermore rejected the simple dichotomy of markets or government failures to explaining lacking innovation success. Instead, they investigated interactions between different actors, their networks and institutions for barriers and blocking mechanisms (see for instance the very generative approach of “innovation systems” (Freeman, 2000; Sharif, 2006). STS scholars, on the other hand, emphasized that technologies are socially constructed, creating seamless webs of interrelated artifacts, such as technologies and their environment of firms, banks, regulators and users, which facilitate certain trajectories for technological development while hampering others (Hughes, 1983; Hughes, 1987).
Building on these antecedents, key frameworks in transition studies – e.g. the multi-level perspective on transitions (MLP), technological innovation systems (TIS) or Strategic Niche Management (SNM) – adopted this configurational logic to understanding and explaining the unfolding of innovation and transformation processes (Geels, 2022). Unlike in mechanistic approaches, where sets of – at best mutually independent – factors explain the relevant outcome (here innovation success, or successful sector transformations), configurational explanation emphasizes that (interdependent) configurations of factors are critical for explaining the inner workings and outcome of socio-technical transformation processes (Abbott, 2001; Furnari et al., 2021; Goertz and Mahoney, 2012). In this logic, socio-technical systems underpinning sectors such as energy, water and transport, are often seen as evolving in a path-dependent manner. Dominant solutions like car-based mobility or fossil-fuel based energy are typically conceptualized as “configurations that work” (Rip and Kemp, 1998), i.e. highly institutionalized configurations of actors, technologies and institutions that remain stable over long time spans (Kemp, 1994; Rip and Kemp, 1998). The related set of core rules, i.e. the socio-technical regime leads incumbent actors to favor incremental over radical innovations and by this the socio-technical systems tend to exhibit strong path dependencies. Accordingly, socio-technical transitions can be conceptualized as reconfigurations of the alignments among actors, technologies and institutions from one established configuration towards one or several new ones (Rip and Kemp, 1998; Markard et al., 2009). As there is a confusion in terms in much of the transitions literature about the concepts of socio-technical regimes and socio-technical systems (Geels, 2011), we declare here that the material configuration of actors, technologies and institutions will be called a socio-technical system, while the term “regime” is reserved for the core rule sets (i.e. the highly institutionalized rules, norms and regulations), which shape the actions and strategies of incumbent actors (for a more explicit discussion, see Fuenfschilling and Truffer, 2014).
A second key inroad for understanding transitions relates to exploring how new, socio-technical configurations emerge, diffuse and scale. Relevant frameworks like technological innovation systems (TIS) or strategic niche management (SNM) also typically adopt a configurational lens, yet with a stronger focus on the early, still fluid and emergent development phases of technologies and/or industries. Especially in TIS studies, the build-up of coherent configurations of interrelated innovation structures (actors, networks, institutions) and the prevalent processes (so-called functions) serve to explain the success or failure of innovations (Hekkert et al., 2007; Suurs and Hekkert, 2009). Ultimately, STCA can be used to analyze both how dominant regime configurations emerge, get stabilized, reproduced or dismantled over time and space, as well as for understanding how and where innovation systems emerge, how they “mature” and how they gradually reconfigure or transform incumbent socio-technical systems.
2.1 Measuring and mapping socio-technical configurations
Given the configurational “ontology” implicitly adopted by most socio-technical transitions research, the question remains on how an appropriate epistemology could look like that enables the systematic tracking of the evolution of socio-technical configurations over space and time. Up until now, most transitions research built on rich qualitative case studies and historical reconstructions of socio-technical transformations (Zolfagharian et al., 2019). In this line of work, relevant configurations are identified by knowledgeable analysts, who are able to identify the most important relationships between actors, technologies and institutional elements through absorbing and interpreting information from a broad range of documents, as well from interviews, focus groups, action research, etc. (Geels and Turnheim, 2022). A broadly accepted weakness of this approach is, however, that the analytical procedures are hard to replicate and results often are relatively difficult to generalize beyond the single case studies analyzed.
STCA addresses this weakness by proposing a more standardized, semi-quantitative approach, which follows earlier approaches of text, event or discourse analysis, but combines them with social network analysis thinking. By doing so, it allows for a systematic identification of how socio-technical configurations emerge, dissolve and get transformed over time. In transition studies, this often relates to changes in specific technological sectors promising to resolve a particular sustainability problem. However, STCA as a methodological approach is not limited to assessing technology dynamics and does not require any particular normative motivation, like addressing sustainability issues or grand challenges. In principle, any configuration of concepts could be analyzed with it. However, in the following, we will mostly focus on its application range within transition studies, but also some initial examples of our recent work on how it can be extended to research questions in economic geography.
An important methodological step before embarking on an STCA relates to choosing an appropriate stock of documents. The STCA analyst has to be aware of the way in which actor’s framings of technologies and rules or of actual events will be represented in the text data. We therefore need a sort of “theory of measurement” for each type of document stock (Goertz, 2020), i.e. how the “data” recorded in the text relate to the actual phenomenon that the researcher is interested in and what kinds of translational steps intervene in this process. Newspapers, for instance, only report on aspects that seem newsworthy for their specific readership. Newsrooms will pro-actively filter events and issues and therefore provide a selective view on the socio-technical dynamic in focus. More specifically, news articles will only report on “interesting” (for a target audience at a particular point in time) features and will not elaborate too deeply on taken-for-granted elements in a system.
Therefore, we might expect only selective configurational relationships to appear in public discourse in normal times. Only in critical moments, when crises occur or a sector is facing strong endogenous development challenges, actors will be forced to be more outspoken about the rationale of their proposals on how to best confront the imminent challenges, or why radical innovation is needed (Yuana et al., 2020; Rosenbloom, 2018). For instance, in the wake of climate change, a nuclear power company would probably present its technology as a preferable and unproblematic means to save greenhouse gas emissions that just needs supportive context conditions for its operation. Environmental NGOs will instead comment on problems of nuclear waste and promote renewable energies as the go-to solution for the future, due to decreasing costs and lower environmental and social externalities. These articulations may be direct, for instance when expressed in the context of an expert interview, a press release, or a public hearing. Alternatively, they may be presented indirectly when journalists write about the different positions of actors. Understanding the specific information filtering and translational processes applied in the different document stocks is therefore key for correctly interpreting STCA results.
Once the document stock is determined and the relevant texts are selected from it (see Chapter 2), the actual STCA coding procedure can be summarized as follows: Coding focuses on identifying actors, and the “concepts” that these actors address in their discourses and actions. We will use the word “concepts” here as a short-cut for all non-actor aspects that will be coded in view of a specific research question: technologies, values, norms, practices, policies etc. In the coding process, the analyst will identify words, terms, expressions, arguments and aggregate them into coherent codes, which should resonate with the phenomenon studied, as well as with the conceptual framework used. In other words, concepts connect the empirical data with conceptual frameworks (see Chapter 3). They therefore have similarity to what quantitative analysts would call “factors” or “variables”.
In other words, concepts in STCA cover all potential components of socio-technical configurations, thus encompassing a broad set of ‘material’ elements, like technologies, infrastructures, or artefacts, as well as institutional aspects like modes of governance, values, norms, cultural beliefs, rules, routines, practices, and so on. The configurational aspect will be derived from how two concepts get associated through statements or actions of specific actors – or from how actors get associated through shared narratives or actions. An associative ‘link’ between two concepts (or two actors) is typically derived from their co-appearance in statements or actions of particular actors (or the joint reference to a concept by two, or several actors). Aggregating across the whole set of analyzed documents, these individual connections may then be added into overarching “similarity” scores that represent the salience of two concepts in the broader technological field (see Chapter 5-7). Clusters of strongly related concepts may then be interpreted as dominant or peripheral storylines in a field, depending on the number and type of actors that support them (Simoens et al., 2022). And these storylines may serve as proxies for more or less coherent socio-technical configurations that exist in the field (ibid.). The structure, dominance and coherence of these configurations can then be compared among different case studies, sectors, as well as across space and time and be used for various forms of (configurational) theorizing. In more technical terms, the procedural steps will be executed as follows: Concepts and actors will be typically identified by means of a qualitative and recursive coding process using a conventional qualitative coding software (Nvivo, MaxQDA or similar, see Chapter 3). Overlaps of codes will be exported as co-occurrence matrices from these software programs and then be further transformed (e.g. by means of R-scripts), into association or similarity matrices between actors or concepts. The associations or similarities may then be represented in different types of network graphs (see fig. 1). The most direct representation of these data is in so-called two-mode networks, where actors and concepts are presented as directly linked nodes, indicating which actors used which concepts (i.e. represented by the black arrows within the dotted box). This relational information can be further condensed into so-called one-mode networks of two types: Either links between actors can be defined based on their reference to a shared concept (see red arrows between both actors a1 and a3 to the concept c1 represented by a thick dotted red line between the two actors). This results in a one-mode actor congruence network, which draws actors closer together who share many concepts and separates those that have little overlap in their actions and statements. The same original two-mode network may be used to aggregate a one-mode concept network. Concepts will be linked based on their co-mentioning by different actors. Resulting networks will therefore depict clusters of concepts, which have been co-mentioned by groups of actors. See for instance the thick, green, dotted line between c4 and c5 which resulted from statements made by a4.
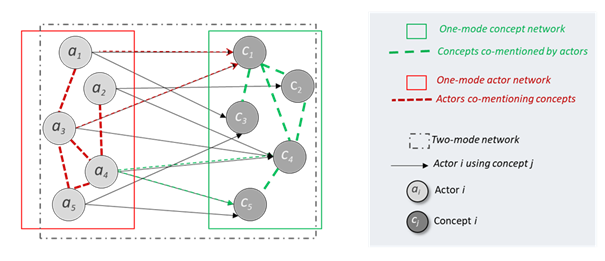
This approach allows mapping socio-technical configurations in a specific (most often technological or sectoral) field. In many applications of STCA, researchers are less interested in personal views and attitudes of particular individuals. Coded statements by individuals are rather taken as representative of their respective organizations, which through their statements and actions express their position in the respective field. The actor congruence networks, in this logic, represent ideational similarities among different actor groups presenting narratives that support specific socio-technical configurations. In order to facilitate the interpretation of the derived networks, often additional variables from the dataset may be used to increase their readability, like actor types, number of times coded, location, type of relationships, etc. These may be represented by varying sizes, forms or colors of nodes and edges in the graphs. Additionally, network indicators can be calculated to identify a configuration’s maturation or degree of institutionalization, as well as the degree of coherence and/or stability of the various configurations that co-exist in a given field (see Chapter 6-7). Ultimately, transition scholars will often be interested in tracing the evolution of configurations over time or the variation of configurations across space.
3. A typology of research problems to be tackled by STCA
STCA opens up many different inroads for analyzing socio-technical reconfiguration dynamics. In the context of transitions studies, the method will be most often applied to tracing how social and technical elements get aligned (or de-aligned) and/or on how incumbent socio-technical configurations get transformed over time and space (Rip and Kemp, 1998). In the remainder, we thus focus our elaborations on this specific application field. Later, we will also quickly explore application potentials in the field of economic geography.
3.1 Types of variables to generate networks in STCA
In its standard application, STCA aims at mapping socio-technical configurations by coding information from a stock of documents. As we will see any type of code or attributes (concepts, actors, temporal and spatial references, etc.) can assume different “roles”, depending on the phenomenon to be mapped. In order to construct a preliminary typology of the different kinds of research questions that may be tackled with STCA, we have to first introduce different roles that codes may play in STCA giving rise to particular graphical representations of the observed phenomenon (i.e. the configurations in focus). We will call codes that assume a specific role as different kinds of “variables” in the following, because they emphasize specific aspects that are foundational to answer a specific research question. To illustrate why we need to distinguish between types and roles of variables, think of a conventional multivariate regression analysis aiming to explain a specific outcome. Here variables will essentially be distinguished into “dependent” and “independent” variables, where the former relate to the phenomenon to be explained and the latter to the different factors associated with the occurrence of the phenomenon Yet, in contrast to regression analysis, in an STCA logic, variables are not seen as independent from each other, but as jointly creating a certain outcome (or not).
We will here present four different roles that variables can take on in STCA. The first two roles apply especially to the construction of one-mode concept or actor congruence networks. The third and fourth also apply to two-mode networks.
First, ‘mapped variable(s)’ are the codes that will be represented by nodes in a one-mode network graph. They thus relate to those codes that will be represented by nodes exhibiting higher or lower vicinity/similarity among each other. In the case of analyzing shifts in socio-technical configurations, the mapped variables typically relate to concepts (technologies, values, regulations, institutions) or any combination of these codes. In the example of Heiberg et al. (2022) the mapped variables comprise technologies, governance arrangements, paradigms, and financial incentives that together allow inferring coherent socio-technical configurations in the water sector.
In order to determine the proximity, strength of alignment or similarity among the mapped nodes, we need at least a second key (set of) code(s), which we call the ‘associating variable(s)’. This variable could for instance be actors that consistently co-mention concepts in their statements or regularly combine the two concepts in innovation activities. In the case of Heiberg et al. (2022) and (Yap et al., under revision), the associating variable are organizations (firms, research institutes, government agencies etc.) that voice their opinion about certain solutions to water or space debris problems in public media. In other cases, associating variables could also be scientific papers (Truffer et al., 2022), project reports (Lesch, 2022), technologies, or core values / institutional logics (Heiberg and Truffer, 2022b).
While mapped and associating variables are necessary for the construction of one-mode concept or actor congruence networks, one may typically also want to add partitioning variables, which enable creating networks for separate sub-sets of the full database in order to compare configurations across, space, time, etc. Probably the most obvious partitioning variable is time, e.g. when specific development phases can be identified, between which major configurational shifts took place (Heiberg et al., 2022; Yap et al., forthcoming). Separate networks may then be drawn for each time period in order to retrace the respective transition trajectory. In a similar vein, geographical location may serve as a partitioning variable, enabling comparisons of socio-technical configurations across different regions, countries or scales (Heiberg et al., 2022; Heiberg et al., 2020). Another example of a partitioning variable would be an a priori classification of technologies into ‘regime’ or ‘niche’ solutions (Miörner et al., 2022) or of actors into actor types (firm, research, public, etc.). In yet other cases, partitioning the data may be based on sentiments (i.e. actors support for or opposition against certain mapped variables (Miörner et al., 2022). It is also possible to structure the data using differences between document stocks such as newspaper articles and project reports as a partitioning variable. Obviously, all these examples may also be applied in combinations, as in the case of comparing configurational dynamics over time and across space in Heiberg et al. (2022). Finally, the researcher might want to further include ‘complementary variables’ to improve the information content in network graphs and their comparability. Typical examples are types of actors (e.g. determined by clustering algorithms, or a priori classifications), number of times a specific concept or actor has been coded, similarity scores between concepts, aggregate centrality of each concept or actor in the field, but also types of technologies, policies, institutional logics, etc. Essentially any code or indicator derived from the code database might add relevant information to the networks regarding size, color or shape of nodes and links.
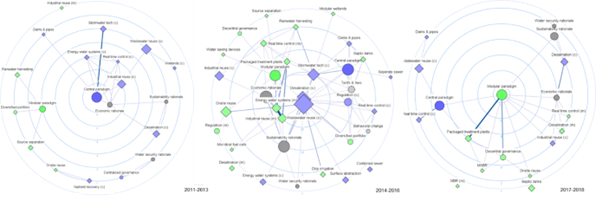
Shift in the discourses about solutions to water related problems in the USA between 2011 – 2018, divided into three development phases representing the period of major drought (2014-2016) as well as one period before and after. The mapped variables are technologies and institutional codes. The associating variable is given by actors. The partitioning variable is given by the three time periods. A number of complementary variables have been added: Blue nodes represent concepts emphasized by regime actors favoring conventional large-scale infrastructures, while green nodes are concepts pushed by ‘niche’ actors favoring small-scale water reuse technologies. Diamond shapes depict technical concepts, circles institutional ones. Thickness of the links between concepts is proportional to their similarity score. The maps are furthermore structured as “radar plots” i.e. putting the concepts with the highest degree centrality into the center and those with low scores to the periphery. Overall, the nodes are arranged in a way to pull them closer in case of high similarity and farther away in case of lower scores.
3.2 A preliminary typology of STCA applications
Almost any code or combination of codes could potentially take on any of the four roles listed above. The permutations of the codes and roles therefore gives rise to a staggering variety of potential networks that can be drawn out of any specific STCA code database. Which one to choose depends on the specific research interests. In the following, we will sketch out a first typology of applications for STCA spanning socio-technical transitions and economic geography (see table 1).
Type 1 is perhaps the most relevant one for transition studies as it focuses on configurational shifts in the concept and actor congruence networks within a specific socio-technical system. Examples are the analysis of niche-regime dynamics (Heiberg et al. 2022), regime reconfiguration pathways, the maturation of TISs (Heiberg and Truffer, 2022b) or niches, technology invasion and diffusion in specific places (Miörner et al., 2022), or the emergence of regime structures in a novel technological field (Yap et al., under revision). These questions can typically be analyzed by choosing technology and/or institution codes as mapped variables and actors as the associating variable. Partitioning variables may be chosen depending on the specific research question in focus.
Type 2 focuses on the analysis of actor coalitions and directionality in a socio-technical system, asking which actor groups support the development of specific socio-technical configurations based on their guiding values and how this will impact the ‘directionality’ of developments in a field (Yap and Truffer, 2019). In this case, the associating variables are institutions, while actors and technologies represent the mapped concepts. This STCA type thus allows analyzing how different guiding values may influence technology and/or industry dynamics in a sector. An illustrative example is a recent analysis of how core values and interests determine whether innovations pursued by specific actors will add up (or not) into an overarching (national) innovation system structure and how value alignments or conflicts influence the drawing of suitable system boundaries (Heiberg and Truffer, 2022b). Another interesting application field would be the analysis of how (or based on which values) actors engage in the formation of proto-regime structures in an emerging technological field like space debris management (Yap et al., under revision).
Type 3 relates to mapping a technological field in terms of different subcomponents or institutional alignments. Here technological variants or infrastructure solutions are mapped by means of the associating variable of actors and/or institutional codes, . One potential application of this analysis type is to identify and characterize system boundaries in a complex technological field like renewable energies and determining whether coherent technological sub-systems exist (e.g. wind power, biomass, wave technologies), which share core characteristics in social and institutional terms. On the one hand, this enables a prospective evaluation of different technology variants in a sector and define generic policy interventions that could support several sectors at once (Markard et al., 2009). Second, the approach could prove instrumental in disentangling ‘institutional relatedness’ between technology options, thus giving policy makers important hints on what sort of ‘green’ technology is more likely to be successfully developed in a given region or country (Carvalho and Vale, 2018). Third, it enables the analysis of interfaces between sectors in ‘multi-system interactions’ and tease out which sectoral structures are more or less likely to be successfully integrated, e.g. in the energy-transport or energy-water-food nexus or in the emergence of meta-infrastructures like in the case of the (outer) space sector (Yap and Truffer, 2022).
So far, we have discussed the three most generic types of STCA analyses that are conceivable based on the possible permutation of our mapped and associating variables based on actors’ statements and activities. The method can however also be applied to configurations among spatial entities (cities, regions, countries, etc.).
Type 4 enables the analysis of proximities between places (regions, countries, cities) that result from their engagement with similar socio-technical configurations. The mapped variable here would be “places”, while the associated variables relate to technical and/or institutional codes. This might result in the identification of similarities in the profiles of places, which suggest a heightened potential for a trans-regional flow of knowledge, resources or people between them, which are necessary, e.g. to build a TIS (Heiberg and Truffer, 2022a). One could also use this approach for identifying spatial subsystems in wider Global Innovation System structure (Binz and Truffer, 2017).
Type 5 complements type 4 as it uses places and scales as associating variables in order to represent the complex spatiality and multi-scalar networks of socio-technical configurations. This type might be highly relevant to depict socio-technical configurations that are supported by actors in a majority of regions – i.e. in the sense of a global regime (Fuenfschilling and Binz, 2018). Depending on the relationships among places and scales, one could also more deeply characterize global production network structures, which support one or another type of configuration. Furthermore, one may also distinguish the scalar configuration of certain actors, institutions or technologies and use this to elaborate on the multi-scalarity of niche and regime structures or to analyze structural couplings among regions within a Global Innovation System.
Type 6, finally, focuses on regional industry dynamics and inter-path relations. It aims to reveal the alignment and causal relationships between new, and existing industrial paths in a given region. The associating variable in such an analysis would be the industrial path, i.e. an identified set of functionally related firms in a confined geographical context , while the mapped variables would be represented by actors, institutions and resources that underpin the respective industrial paths (Hassink et al., 2019). This allows for a nuanced analysis of the socio-technical relationships between different industries co-located in the same region, such as the extent to which industrial paths are related institutionally, the nature of their relationships (Frangenheim et al., 2020), and how these develop over time. In addition, focusing exclusively on institutional infrastructures or institutional logics as the associating variable in such an analysis could also be used to reveal the institutional relatedness (Carvalho and Vale, 2018) between different regional industrial activities beyond what has been possible using conventional methodologies.
Table 1: Types of analyses, which may be tackled by STCA.
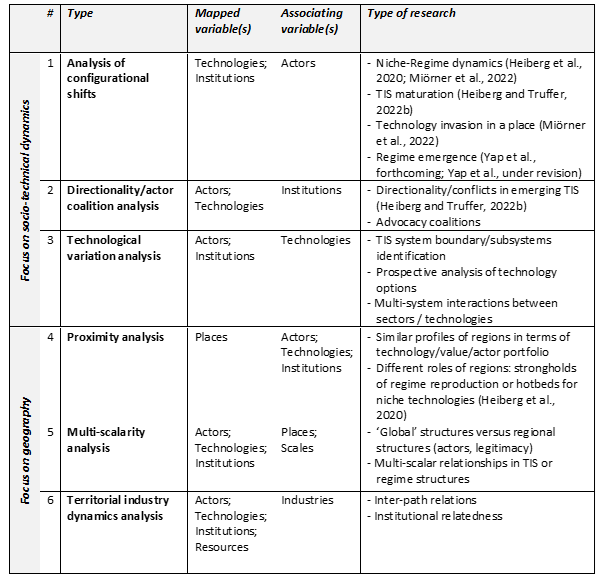
4. Summary and Outlook
The guidebook further elaborates on the concrete procedural steps that a researcher has to consider when applying STCA. After having made sense of the case to be analyzed and having constructed relevant research questions and guiding hypotheses/propositions, there are five steps that are crucial for running an STCA: i) collection of suitable document stocks, ii) coding the selected texts, iii) deriving similarity measures for the construction of two-mode actor-concept networks, as well as for deriving one-mode actor or concept networks and iv) optimizing the network representation for the analytical tasks at hand.
Depending on the research problem envisaged, further analytical steps can of course be added such as clustering analysis or other subgroup identification algorithms, applying various quantitative SNA indicators for an in-depth exploration of the network structures, doing statistical modelling and comparisons of different networks, comparing STCA maps from different case studies with qualitative comparative analysis, etc. Also supplementing STCA analysis with expert interviews has proven a powerful approach for identifying the causal mechanism that explain the (re)configuration dynamics that emerge from the network analysis.
At its current development stage, STCA is primarily a method for identifying and mapping socio-technical configurations and tracing their evolution over space and time. In order to proceed from description and exploration to explanation, it has to be complemented with process tracing and other qualitative content analysis approaches or with quantitative methods in case network indicators (such as centrality, density, modularity, etc.) are used as explanatory variables. The major contribution of STCA is that it enables systematic and transparent way to retrace shifts in socio-technical configurations. In that sense it enables comparative research across cases, time periods and geographies, which may in turn enable a more standardized and cross-comparative type of theorizing in transition studies and related social sciences. The guidebook represents the current state of method development, but of course acknowledges the tremendous further development potentials in all of the above respects. We thus invite others to contribute to this endeavor and contribute to improving the methodology and its theoretical underpinnings.
After this introductory chapter, the following chapters will turn to a more practical level and explain how to carry out each step of an STCA in a rather detailed and illustrative way. The main goal is to enable other researchers to carry out an STCA on their own. The guidebook represents the current state of method development and will be continuously updated, as our collective understanding of the method’s strengths and limitations develops.
References
Abbott, A. (2001) Time matters: On theory and method. University of Chicago Press.
Balland, P.-A., Broekel, T., Diodato, D., Giuliani, E., Hausmann, R., O’Clery, N. and Rigby, D. (2022) ‘The new paradigm of economic complexity’, Research Policy, 51(3), pp. 104450.
Binz, C. and Truffer, B. (2017) ‘Global Innovation Systems – A conceptual framework for innovation dynamics in transnational contexts’, Research Policy, 46, pp. 1284-1298.
Carvalho, L. and Vale, M. (2018) ‘Biotech by bricolage? Agency, institutional relatedness and new path development in peripheral regions’, Cambridge Journal of Regions, Economy and Society, 11(2), pp. 275-295.
Creswell, J. W. (2009) Research Design – Qualitative, Quantitative and Mixed Methods Approaches. 3rd edn. Thousand Oaks, CA: Sage Publications.
Dosi, G., Freeman, C., Nelson, R., Silverberg, G. and Soete, L. (1988) Technical Change and Economic Theory. London: Pinter.
Frangenheim, A., Trippl, M. and Chlebna, C. (2020) ‘Beyond the Single Path View: Interpath Dynamics in Regional Contexts’, Economic Geography, 96(1), pp. 31-51.
Freeman, C. (2000) ‘The ‘National System of Innovation’ in historical perspective’, in Edquist, C. and McKelvey, M. (eds.) Systems of Innovation: Growth, Competitiveness and Employment. Cheltenham: Edward Elgar, pp. 41-60.
Fuenfschilling, L. and Binz, C. (2018) ‘Global socio-technical regimes’, Research Policy, 47(4), pp. 735-749.
Fuenfschilling, L. and Truffer, B. (2014) ‘The structuration of socio-technical regimes—Conceptual foundations from institutional theory’, Research Policy, 43(4), pp. 772-791.
Furnari, S., Crilly, D., Misangyi, V. F., Greckhamer, T., Fiss, P. C. and Aguilera, R. V. (2021) ‘Capturing causal complexity: Heuristics for configurational theorizing’, Academy of Management Review, 46(4), pp. 778-799.
Geels, F. W. (2011) ‘The multi-level perspective on sustainability transitions: Responses to seven criticisms’, Environmental Innovation and Societal Transitions, 1(1), pp. 24-40.
Geels, F. W. (2022) ‘Causality and explanation in socio-technical transitions research: Mobilising epistemological insights from the wider social sciences’, Research Policy, 51(6), pp. 104537.
Geels, F. W. and Turnheim, B. (2022) The Great Reconfiguration. Cambridge University Press.
Goertz, G. (2020) Social science concepts and measurement: New and completely revised edition. Princeton University Press.
Goertz, G. and Mahoney, J. (2012) A tale of two cultures. Princeton University Press.
Hajer, M. A. (1995) The Politics of Environmental Discourse : Ecological Modernization and the Policy Process. Oxford: Clarendon Press.
Hassink, R., Isaksen, A. and Trippl, M. (2019) ‘Towards a comprehensive understanding of new regional industrial path development’, Regional Studies, 53(11), pp. 1636-1645.
Heiberg, J. (2022) The Geography of Configurations that Work: An inquiry into sustainability transitions and industry formation in the water sector. Ph.D., Utrecht University, Utrecht.
Heiberg, J., Binz, C. and Truffer, B. (2020) ‘The Geography of Technology Legitimation. How multi-scalar legitimation processes matter for path creation in emerging industries’, Economic Geography, 96(5), pp. 470-498.
Heiberg, J. and Truffer, B. (2022a) ‘The emergence of a global innovation system – A case study from the urban water sector’, Environmental Innovation and Societal Transitions, 43, pp. 270-288.
Heiberg, J. and Truffer, B. (2022b) ‘Overcoming the harmony fallacy: How values shape the course of innovation systems’, Environmental Innovation and Societal Transitions, 42, pp. 411-428.
Heiberg, J., Truffer, B. and Binz, C. (2022) ‘Assessing transitions through socio-technical configuration analysis – a methodological framework and a case study from the water sector’, Research Policy, 51(1), pp. 104363.
Hekkert, M., Suurs, R., Negro, S., Kuhlmann, S. and Smits, R. (2007) ‘Functions of innovation systems: A new approach for analysing technological change’, Technological Forecasting and Social Change, 74(4), pp. 413-432.
Hughes, T. P. (1983) Networks of power: electrification in Western society, 1880-1930. Baltimore: John Hopkins University press.
Hughes, T. P. (1987) The evolution of technological systems. The Social construction of technological systems Camebridge, Mass.: MIT Press.
Kemp, R. (1994) ‘Technology and the transition to environmental sustainability: The problem of technological regime shifts’, Futures, 26(10), pp. 1023-1046.
Leifeld, P. (2013) ‘Reconceptualizing Major Policy Change in the Advocacy Coalition Framework: A Discourse Network Analysis of German Pension Politics’, Policy Studies Journal, 41(1), pp. 169-198.
Lesch, D. (2022) The Role of Global Actors in Sustainability Transitions. M.Sc. Master thesis, Lund University, Lund.
Markard, J., Stadelmann, M. and Truffer, B. (2009) ‘Prospective analysis of technological innovation systems: Identifying technological and organizational development options for biogas in Switzerland’, Research Policy, 38(4), pp. 655-667.
Miörner, J., Heiberg, J. and Binz, C. (2022) ‘How global regimes diffuse in space–Explaining a missed transition in San Diego’s water sector’, Environmental Innovation and Societal Transitions, 44, pp. 29-47.
Misa, T. J. (1998) A nation of steel: The making of modern America, 1865-1925. JHU Press.
Mitchell, M. (2009) Complexity: A guided tour. Oxford university press.
Nelson, R. R. and Winter, S. G. (1982) An Evolutionary Theory of Economic Change. Cambridge, Massachusetts, and London, England: Belknap Press of Harvard University Press.
Rip, A. and Kemp, R. (1998) ‘Technological Change’, in Rayner, S. and Malone, E.L. (eds.) Human choice and climate change – Resources and technology. Columbus: Battelle Press, pp. 327-399.
Romer, P. M. (1994) ‘The origins of endogenous growth’, Journal of Economic perspectives, 8(1), pp. 3-22.
Rosenbloom, D. (2018) ‘Framing low-carbon pathways: a discursive analysis of contending storylines surrounding the phase-out of coal-fired power in Ontario’, Environmental innovation and societal transitions, 27, pp. 129-145.
Scott, W. R. (1995) Institutions and Organizations. Thousand Oaks: Sage Publications.
Sharif, N. (2006) ‘Emergence and development of the National Innovation Systems concept’, Research Policy, 35, pp. 745–766.
Simoens, M. C., Fuenfschilling, L. and Leipold, S. (2022) ‘Discursive dynamics and lock-ins in socio-technical systems: an overview and a way forward’, Sustainability Science, pp. 1-13.
Smith, A., Voss, J.-P. and Grin, J. (2010) ‘Innovation studies and sustainability transitions: the allure of the multi-level perspective, and its challenges’, Research Policy, 39(4), pp. 435-448.
Suurs, R. A. A. and Hekkert, M. P. (2009) ‘Cumulative causation in the formation of a technological innovation system: The case of biofuels in the Netherlands’, Technological Forecasting and Social Change, 76(8), pp. 1003-1020.
Truffer, B., Rohracher, H., Kivimaa, P., Raven, R., Alkemade, F., Carvalho, L. and Feola, G. (2022) ‘A perspective on the future of sustainability transitions research’, Environmental Innovation and Societal Transitions, 42, pp. 331-339.
Weber, M. and Truffer, B. (2017) ‘Moving innovation systems research to the next level: towards an integrative agenda’, Oxford Review of Economic Policy, 33(1).
Yap, X.-S., Coyle, A. and Moors, E. (forthcoming) ‘How emergent socio-technical regimes of satellite mega-constellations impact global space governance’, GEIST – Geography of Innovation and Sustainability Transitions – Working Paper series.
Yap, X.-S., Heiberg, J. and Truffer, B. (under revision) ‘Emergent global regime complexes of the space debris challenge: A socio-technical configuration analysis’, Submitted to a peer-reviewed journal.
Yap, X.-S. and Truffer, B. (2019) ‘Shaping selection environments for industrial catch-up and sustainability transitions: A systemic perspective on endogenizing windows of opportunity’, Research Policy, 48(4), pp. 1030-1047.
Yap, X.-S. and Truffer, B. (2022) ‘Contouring ‘earth-space sustainability’’, Environmental Innovation and Societal Transitions, 44, pp. 185-193.
Yuana, S. L., Sengers, F., Boon, W., Hajer, M. A. and Raven, R. (2020) ‘A dramaturgy of critical moments in transition: Understanding the dynamics of conflict in socio-political change’, Environmental Innovation and Societal Transitions, 37, pp. 156-170.
Zolfagharian, M., Walrave, B., Raven, R. and Romme, A. G. L. (2019) ‘Studying transitions: Past, present, and future’, Research Policy, 48(9), pp. 103788.